Presenter
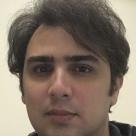
Abstract
With the advent of cloud computing, many different types of our data such as medical records, financial transactions, and access logs are stored at remote servers across the globe. This data is being constantly used by a broad range of applications that perform computation remotely for a variety of purposes, including financial reports, statistical analysis, and business analytics. Many of these applications leverage Machine Learning (ML) algorithms for data analysis and decision making. In such applications, it is critical to hide the identity of the data items used in the computation from the cloud operators. We refer to this notion of privacy as data access privacy. The data access privacy provides guarantees to the data owners that the cloud provider does not know whether their data is used by various computing applications. We refer to the process of computation with private data access as private computation. The objective of private computation is to minimize the amount of data that needs to be downloaded from the remote provider(s) while guaranteeing data access privacy. Private computation generalizes classical Private Information Retrieval (PIR) problem by enabling the applications to perform remote computation on the data, and is generally more efficient than first retrieving data privately (using a PIR scheme) and then performing computation on the retrieved data.
Private computation is a fascinating topic with many breakthrough results reported over the recent years by the information and coding theory community. That said, many interesting problems in this field remain open, providing a fertile ground for future research. The goal of this tutorial is to provide a comprehensive survey of the broad range of private computation problems. We discuss both the state-of-the-art achievability schemes and converse proof techniques as well as open research problems. First, we will discuss the Private Linear Computation (PLC) problem and the Private Linear Transformation (PLT) problem whose goal is to compute a single linear combination and multiple linear combinations of a subset of data items, respectively. These problems are motivated by several ML applications including a linear transformation for dimensionality reduction and training linear models for regression or classification. We will discuss several variations of the PLC and PLT problems, which include different types of data access privacy, the presence of side information, the single-provider and multiple-provider scenarios, and the presence of colluding providers. We will also discuss the general private computation scenarios and the related open problems.